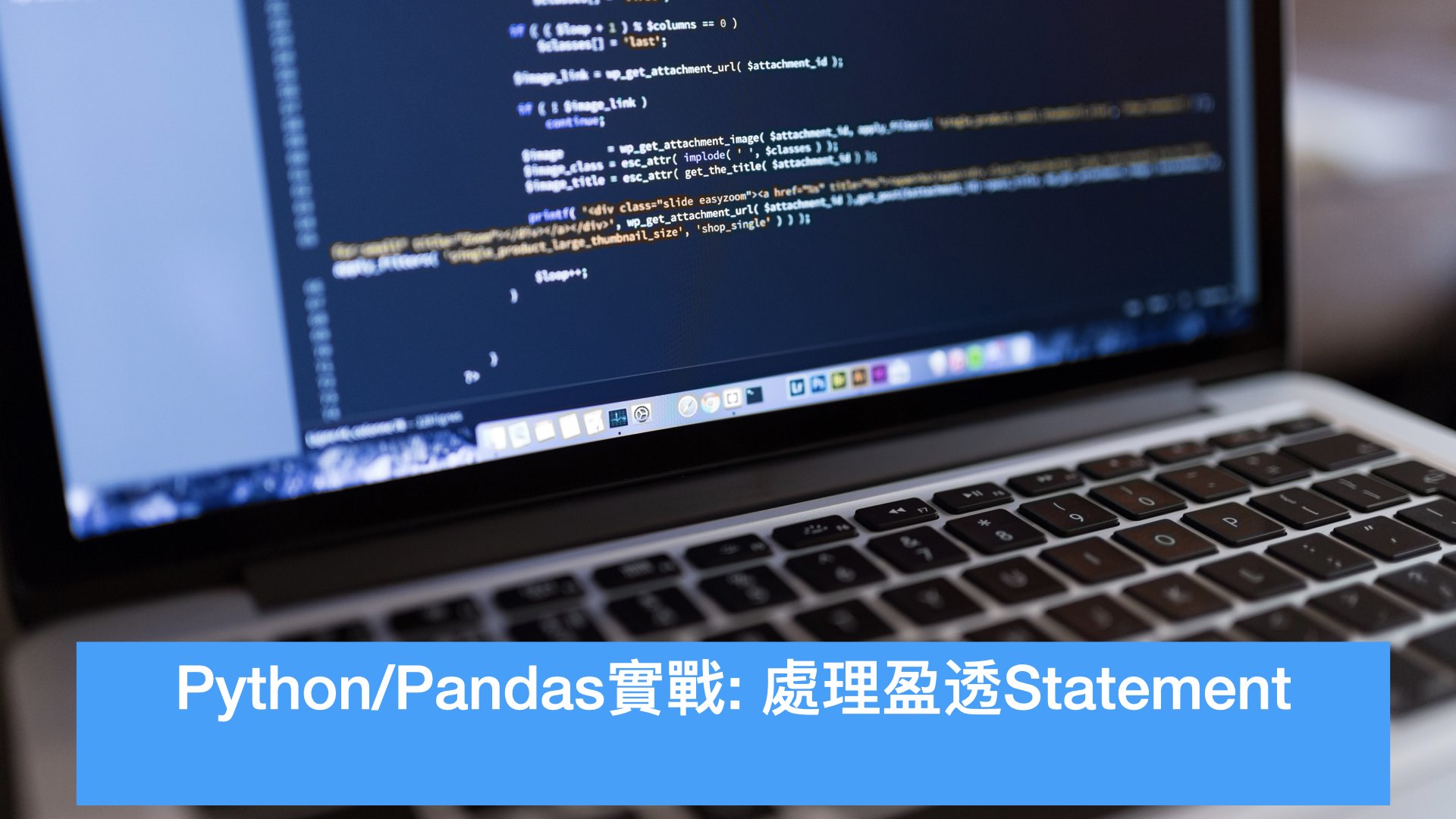
Pandas是一个非常强大的数据分析方面的Python package. 如果是做Machine Learning或者数据分析, 掌握Pandas很省去很多麻烦. 许多Machine Learning前期的数据处理也是用Pandas做得.
IBKR(Interactive Brokers, 有时简称IB, 中文叫盈透证券)是美国老牌券商, 也是我的主要使用的券商. 又到了辞旧迎新的时候, 需要看看2020投资收益, 于是趁新年长周末写点小程序做点数据分析. 而这正好覆盖了Pandas的各种常用functions.
下载IB Statement
IB Statement提供多种方式下载, 比如html, pdf, csv. 用作数据处理选csv. 内容大概长这样
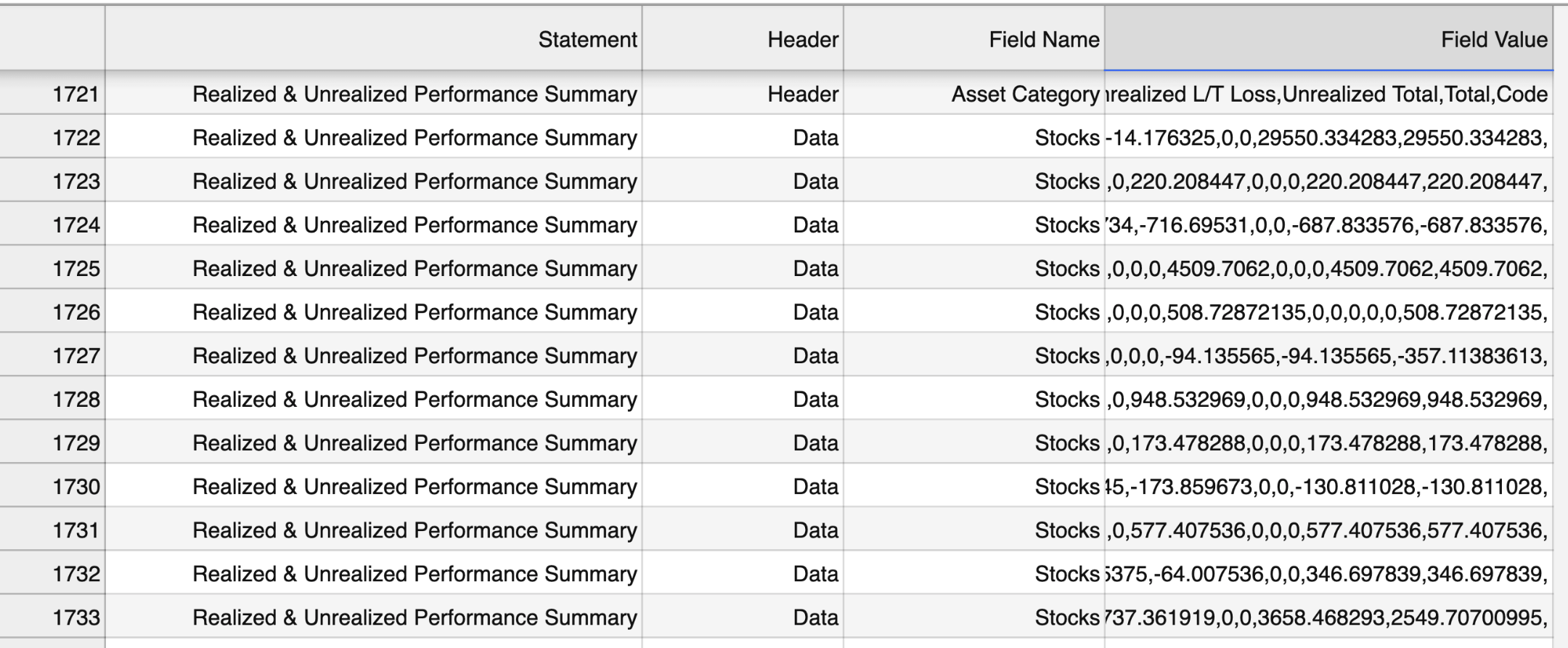
Statement这个column是内容, 里面有很多项, 而这里我只看“Realized & Unrealized Performance Summary”, 然后相同的第一列后面的列数都是一样的. 上图是Jupyter Lab的显示有问题. 因为文件其实可以看成很多CSV files连在一起, 而第一列可以看成是小csv的文件名. 然后第二列是Header或者Data. Header那行就是告诉你后面的Data行里每一列都是什么. 于是我们可以把数据读到内存里.
建立Pandas DataFrame
import pandas as pd field = 'Realized & Unrealized Performance Summary' f = open('statement.csv', 'r') rows = [] for line in f: cols = line.strip().split(',') if cols[0] == field: if cols[1] == 'Header': header_row = cols[2:] else: rows.append(cols[2:])
header_ros是个list of string
['Asset Category', 'Symbol', 'Cost Adj.', 'Realized S/T Profit', 'Realized S/T Loss', 'Realized L/T Profit', 'Realized L/T Loss', 'Realized Total', 'Unrealized S/T Profit', 'Unrealized S/T Loss', 'Unrealized L/T Profit', 'Unrealized L/T Loss', 'Unrealized Total', 'Total', 'Code']
而rows是list of list. 就是list of rows
[['Stocks', 'AAPL', '0', '0', '0', '0', '0', '0', '29564.510608', '-14.176325', '0', '0', '29550.334283', '29550.334283', '\n'], ['Stocks', 'AMD', '0', '0', '0', '0', '0', '0', '220.208447', '0', '0', '0', '220.208447', '220.208447', '\n'], ... ]
接下来就可以用Panda create dataframe了
df = pd.DataFrame(rows, columns = header_row) df.head(5)

这里有个问题. Created出来的dataframe的数据类型有数字有string, 全部都是object. 如果sort by数字column得到的结果是sort by string, 并不是我们想要的. 可以用dtypes来确定
df.dtypes
Asset Category object Symbol object Cost Adj. object Realized S/T Profit object Realized S/T Loss object Realized L/T Profit object Realized L/T Loss object Realized Total object Unrealized S/T Profit object Unrealized S/T Loss object Unrealized L/T Profit object Unrealized L/T Loss object Unrealized Total object Total object Code object dtype: object
这里我把需要转换成数字的columns转换了
header_text_col = set(['Asset Category', 'Symbol', 'Code']) num_col = list(set(header_row) - header_text_col) df[num_col] = df[num_col].apply(pd.to_numeric) df.dtypes
Asset Category object Symbol object Cost Adj. int64 Realized S/T Profit float64 Realized S/T Loss float64 Realized L/T Profit int64 Realized L/T Loss float64 Realized Total float64 Unrealized S/T Profit float64 Unrealized S/T Loss float64 Unrealized L/T Profit int64 Unrealized L/T Loss float64 Unrealized Total float64 Total float64 Code object dtype: object
这样就可以对数字类型的column进行排序了, 比如下面指令做2步操作
1. 选出Asset Category是”Stocks”的rows, 相当于SQL里的where, 语法为df[df['Asset Category'] == 'Stock']
, return是另一个filtered好的dataframe
2. 按Realized Total从小到大排序, return也是另一排序好的dataframe
df[df['Asset Category'] == 'Stock'].sort_values(by=['Realized Total'], ascending=True)
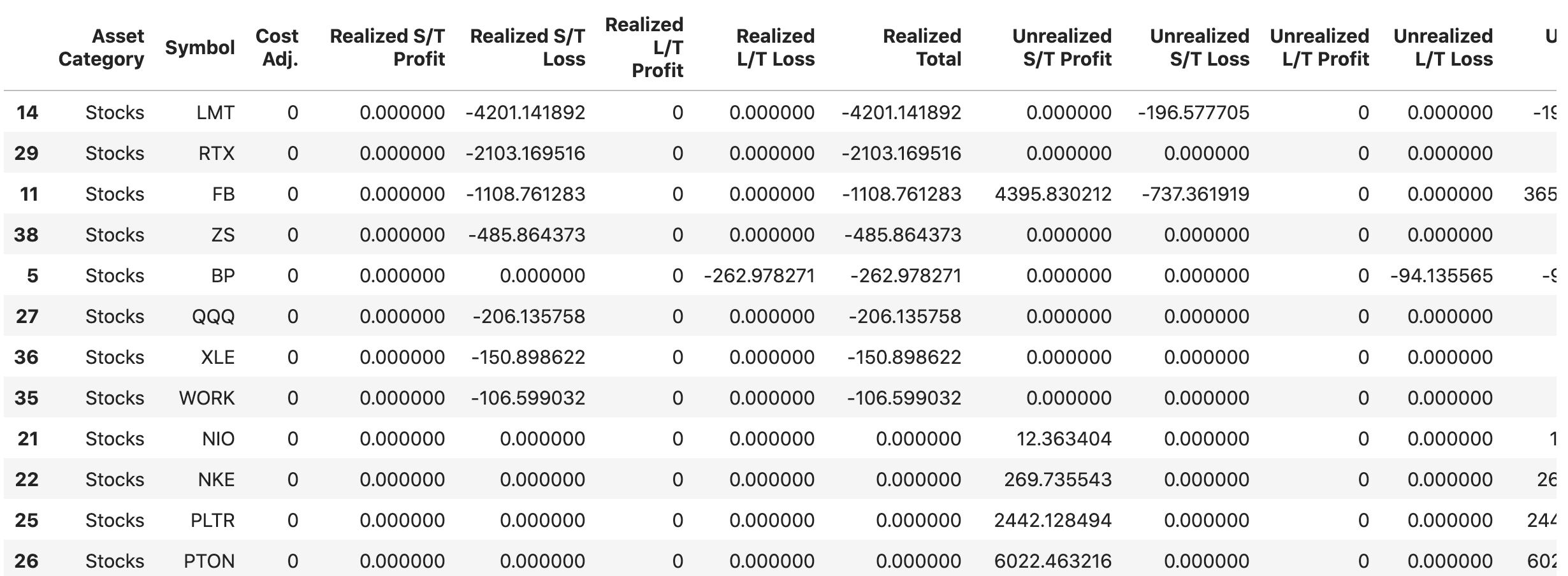
还可以画图
stock_gain = df[ (df['Asset Category'] == 'Stocks') & ( df['Realized Total'] != 0.0)][['Symbol', 'Realized Total']].sort_values( by=['Realized Total'], ascending=True) stock_gain
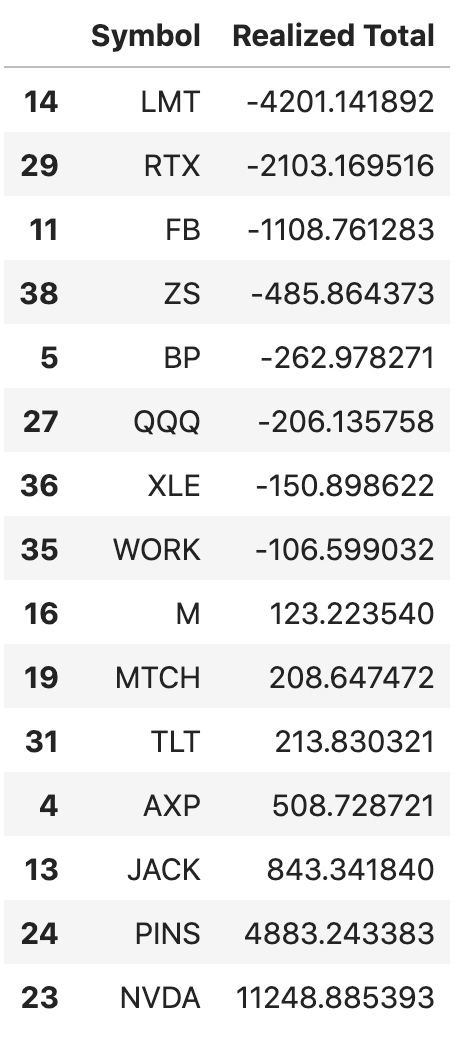
还可以画图
stock_gain.plot.bar(x='Symbol')
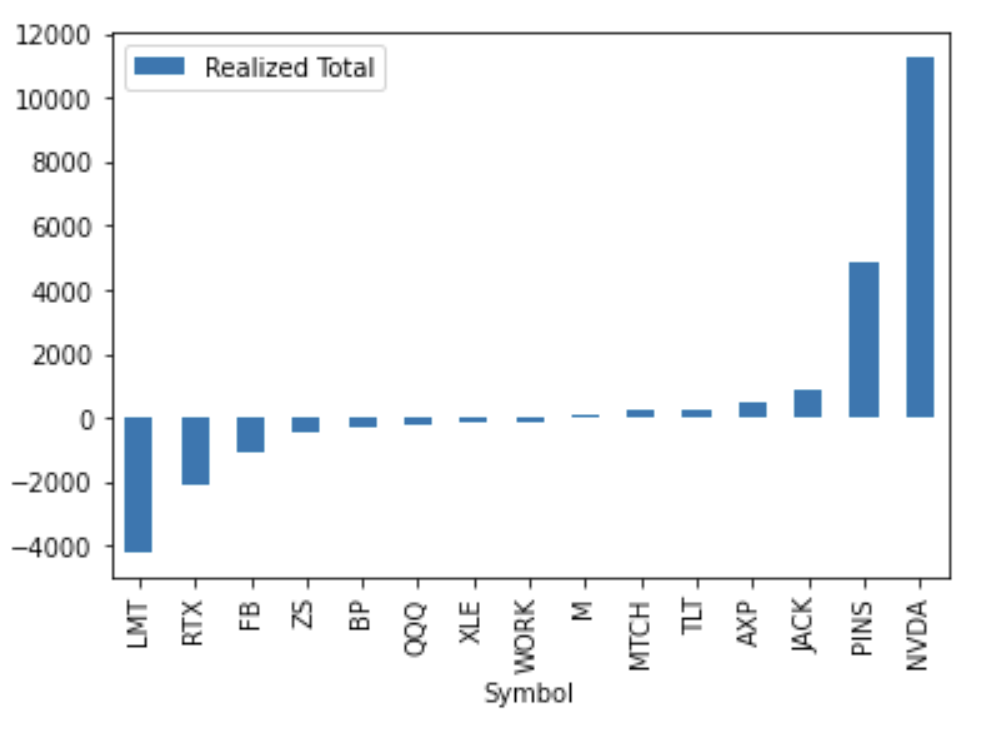
技术小结
建立dataframe
rows是list of rows(lists), header_row是list of string
df = pd.DataFrame(rows, columns = header_row)
显示前n行
df.head(10)
显示后面n行
df.tail(10)
把特定columns转换成数字(numerical type)
num_col是list of strings, 下面命令会把num_col里的columns都转化成数字类. 类似SQL里的select cast(col1 as double), cast(col2 as double), ... from df
df[num_col] = df[num_col].apply(pd.to_numeric)
Filter和sort
类似SQL里, select * from df where asset_category = 'Stocks' order by realized_total asc;
df[df['Asset Category'] == 'Stocks'].sort_values(by=['Realized Total'], ascending=True)